Table of Contents
The most recent iteration of an algorithm called AlphaFold 3, which was made public on May 9, is intended to forecast protein structure, the molecular structures of life with astonishing precisions—essential molecules that are utilized by all living things—from the “instruction code” found in their building parts.
The molecular structures of life with astonishing precision.
One of the main issues in biology has been predicting the shapes of proteins and how they interact with other molecules. However, in the previous several years, AI company Google DeepMind has made significant progress towards a solution. The accuracy and functionality of the AI system have been enhanced over the previous iteration.
Like eagerly awaiting the next installment in a video game series, structural biologists and, more recently, chemists have been awaiting its potential. Although it is commonly believed that DNA serves as a living organism’s instruction manual, proteins are the molecules that actually do the majority of the work inside our cells.
Our cells’ ability to monitor the outside environment, combine data from many signals, create new molecules inside the cell, and choose whether to grow or stop growing is all made possible by proteins.
Additionally, proteins give the body the ability to discriminate between itself and outside intruders like viruses and bacteria. Furthermore, the majority of medications that you and I take to treat illness target proteins.
Lego Protein Structure
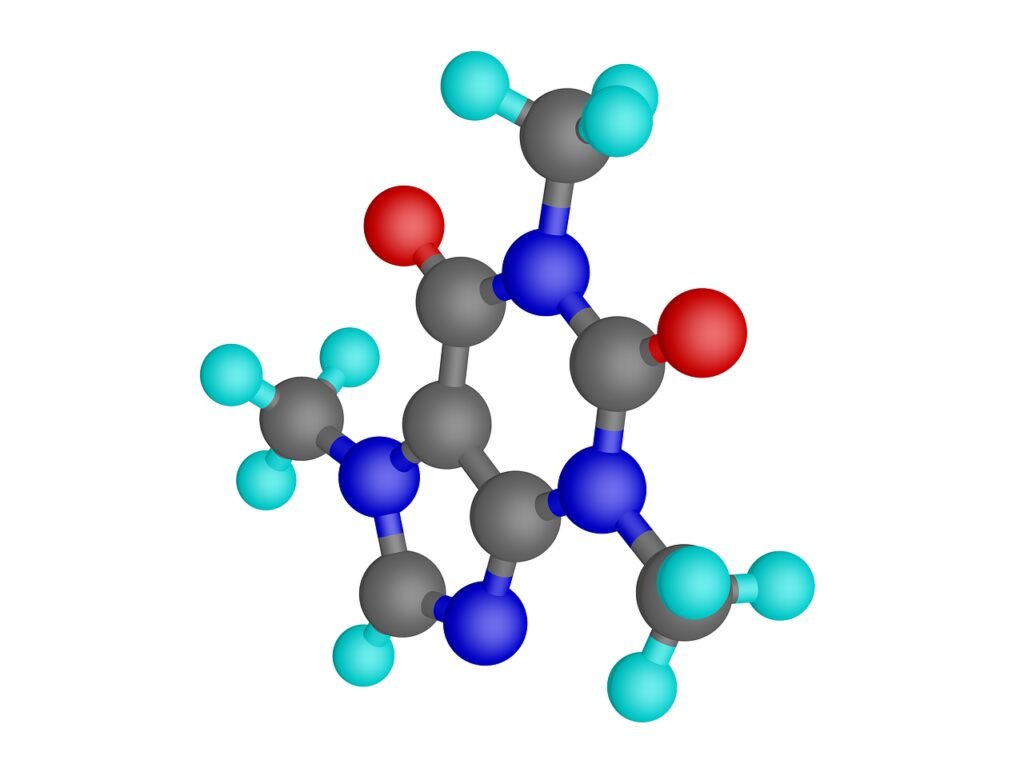
Why is the structure of proteins important? Large molecules with thousands of atoms arranged in precise patterns make up proteins. For a protein to perform its biological job, its atoms must be in the right order and be structured in three dimensions.
The way a medication molecule attaches to its protein target and cures illness is likewise determined by this same three-dimensional structure.
Imagine owning a Lego set where the bricks may be any shape instead of cuboids. Each brick in this set must fit tightly against the other without having any holes in order to be assembled. However, for the bricks to stay in place, the proper arrangement of bumps and holes must also be present in both of the bricks.
Formulating a novel medicinal compound is like constructing a new Lego structure. The task of the drug discovery chemist is to use their toolkit to put together a few bricks that will bind to a specific part of the protein and, in biological terms, prevent it from carrying out its normal function. An enormous model (the protein target found in our cells) has already been built.
What then does AlphaFold perform? AlphaFold is highly good at predicting the 3D structure of every protein based on its knowledge of the precise atoms present in any protein, how these atoms have evolved differently in different species, and the visual characteristics of other protein structures.
The most recent version, AlphaFold 3, has increased capacity to simulate nucleic acids, such as segments of DNA. Additionally, it can predict the morphologies of proteins that have been altered by adding sugar molecules or chemical groups that have the ability to turn a protein on or off. Scientists now have access to more than just a larger, more vibrant Lego set. It implies that they can create more intricate models of cellular regulatory mechanisms and the reading and correction of genetic code.
This is crucial for understanding the molecular mechanisms underlying disease processes and for creating medications that specifically target proteins whose biological function it is to control which genes are active or inactive. Additionally, compared to earlier iterations, the new AlphaFold version predicts antibodies more accurately.
In biology, antibodies are significant proteins that play a fundamental role in the immune system. They are also utilized as biological medications, such as infliximab for rheumatoid arthritis and inflammatory bowel disease and trastuzumab for breast cancer.
The structure of proteins attached to tiny molecules that resemble drugs can be predicted using the most recent version of AlphaFold. If an experiment has determined the 3D structure of the target protein, drug discovery chemists can already anticipate how a prospective drug would bind to it. The process can take months or even years, which is a drawback.
The selection of prospective medications for laboratory synthesis and testing is aided by the prediction of the interactions between possible pharmaceuticals and protein targets. In tests, AlphaFold 3 surpassed previous software predictions, even when the target structure and drug binding site were known. It is capable of predicting drug binding even in the absence of an empirically determined protein structure.
AlphaFold 3 is an intriguing addition to the toolkit used to find novel therapeutic medicines because of these new capabilities. Making better selections regarding which possible medications to test in the lab (and which are unlikely to be beneficial) will be made possible by more accurate forecasts.
Choosing which prospective medications to make and test in the lab depends in part on predicting how potential pharmaceuticals and protein targets bind to one another. In tests, AlphaFold 3 not only beat previous software predictions in the absence of an empirically determined protein structure, but it also performed better in the case where the target structure and drug binding site were known.
With these additional features, AlphaFold 3 is an intriguing addition to the toolkit for finding novel therapeutic medications. It will be easier to decide which possible medications to test in the lab (and which are unlikely to be beneficial) with more accurate forecasts.
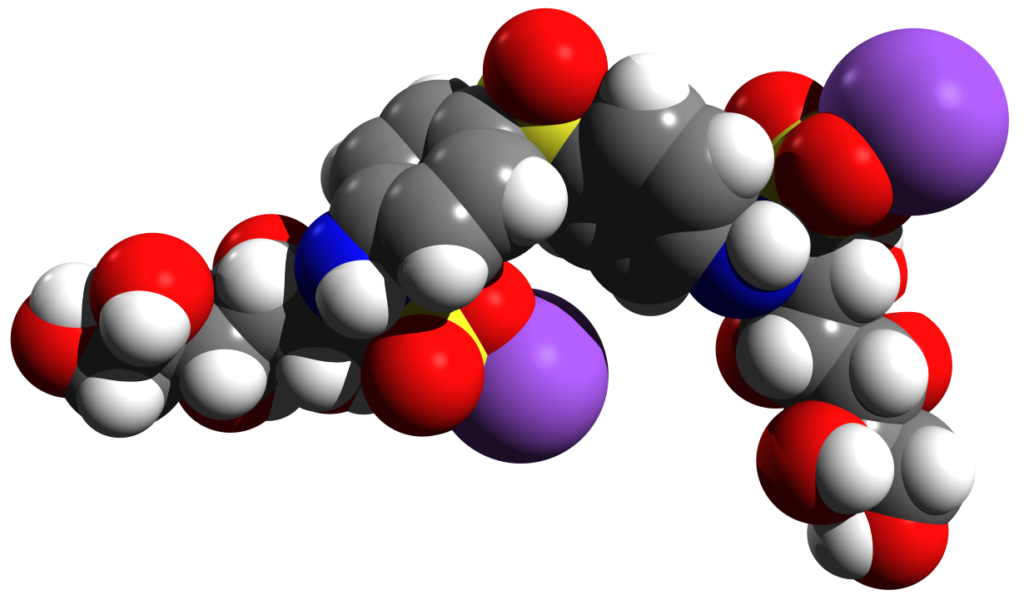
Money and time
Time and money are saved in this way. Additionally, AlphaFold 3 offers the chance to anticipate drug binding to altered protein target forms, which are biologically significant but currently challenging—or impossible—to accomplish with current technologies. Proteins that have had chemical groups like phosphates or sugars added to them are examples of this.
Naturally, before being approved as a licensed medication, a thorough experimental testing program for safety and efficacy—including on human volunteers—is required for any new potential treatment.
AlphaFold 3 is not without its restrictions. It is not very good, like its predecessors, at predicting the behavior of regions of proteins without a fixed or well-ordered structure. It cannot anticipate protein dynamics and does a poor job of forecasting a protein’s many conformations, which can change as a result of drug binding or normal biological processes.
Additionally, it is capable of making a few mildly embarrassing chemical errors, including laying atoms on top of one another, which is physically impossible, and substituting mirror images for certain structural elements, which is impossible both chemically and biologically.
A more significant restriction is that the code will only be available on the DeepMind server for pure non-commercial use as it will be unavailable for the time being. This will limit the excitement of experienced modelers, biotechnologists, and many applications in drug development, but it won’t deter many academic users.
Nevertheless, the introduction of AlphaFold 3 is expected to spark fresh innovation in structural biology in general as well as drug discovery specifically, and we are eagerly anticipating the release of AlphaFold 4.
read also : 8 Horrifying Space-Related Facts
the molecular structures of life with astonishing precision the molecular structures of life with astonishing precision the molecular structures of life with astonishing precision the molecular structures of life with astonishing precision the molecular structures of life with astonishing precision the molecular structures of life with astonishing precision the molecular structures of life with astonishing precision the molecular structures of life with astonishing precision the molecular structures of life with astonishing precision the molecular structures of life with astonishing precision the molecular structures of life with astonishing precision
AI system can predict the structures of life’s molecules with stunning accuracy (msn.com)